How to Write Unit Tests in Python
2021-03-24
用Python进行单元测试
Python编程语言,不仅仅在机器学习、数据分析等领域大放异彩,在web开发中等软件开发中,使用者也越来越多。
在软件开发中,有一种被提倡的开发范式:测试驱动开发。在这种开发范式中,编写单元测试是必不可少的。如果不实施严格的测试驱动开发,编写单元测试程序也是必要的。
对于单元测试而言,最基本的模块是pytest,在本文中会对这个模块给予简要介绍。此外,还有一个现在很流行的模块fizz buzz,本文也会向读者推荐。
为什么要自动化测试
并非所有人都理解自动化测试的必要性,有人甚至认为纯粹是个负担,他们认为自己在编写代码的时候,就已经发现了程序中的BUG,并且已经及时修复了。
这么说,也不是完全没道理。因为我们在开发的时候,就是边写代码、边执行程序,如果有问题,肯定会及时修改。特别是对有丰富开发经验的程序员,编写的代码中错误的确很少。
不过,BUG是难免的。一般情况下,我们都使用已有的框架或者库进行开发,并非从头开始写每一行代码。还有可能是维护、修改、升级原有的功能。在这些情况下,程序中出现BUG的概率就更高了。
因此,自动化测试就不可缺少了。开发者应该将自动化测试视为代码的保险策略,防止由于增加新功能致使BUG产生。
另外一个要实施自动化测试的缘由,是因为人工测试在某些时候是难以完成对程序的所有功能测试。例如,一段程序是从第三方API那个获取一些数据,如果用人工测试,无法测试出对方服务在出现问题是程序获得的异常信息。但是,如果用自动化测试,则能轻易实现。
单元测试、集成测试和功能测试
先简单罗列一下这三种测试的含义:
- 单元测试(Unit tests):又称为模块测试,是针对程序模块(软件设计的最小单位)来进行正确性检验的测试工作。程序单元是应用的最小可测试部件。在过程化编程中,一个单元就是单个程序、函数、过程等;对于面向对象编程,最小单元就是方法,包括基类(超类)、抽象类、或者派生类(子类)中的方法$^{[2]}$。
- 整合测试(Integration tests):又称组装测试,即对程序模块采用一次性或增值方式组装起来,对系统的接口进行正确性检验的测试工作。整合测试一般在单元测试之后、系统测试之前进行。实践表明,有时模块虽然可以单独工作,但是并不能保证组装起来也可以同时工作$^{[3]}$。
- 功能测试(Functional tests):功能测试就是对产品的各功能进行验证,根据功能测试用例,逐项测试,检查产品是否达到用户要求的功能$^{[4]}$。
如你所见,三种测试各司其职。在编写代码是,通常会用单元测试,这个更简单快捷,便于执行。所以,本文仅讨论单元测试。
用Python进行单元测试
Python中的单元测试,就是编写一个测试函数,在其中执行一小段应用程序,检验代码是否正确,如果有问题,会抛出异常。例如,函数forty_two()
返回值是42
,针对这个函数编写的单元测试如下:
1 | from app import forty_two |
这个例子非常简单,在实际的开发过程中会比这复杂,assert
语句也可能不止一条。
要执行这个单元测试,则需将其保存为一个Python文件,然后执行该文件,就能完成测试过程。
Two of the most popular frameworks in Python to write and run unit tests are the unittest package from the Python standard library and the pytest package. For this series of articles I’m going to use a hybrid testing solution that incorporates parts of both packages, as follows:
在Python中有两个非常流行的单元测试框架,一个是标准库中的unittest,另外一个是pytest。
- The object-oriented approach based on the
TestCase
class of theunittest
package will be used to structure and organize the unit tests. - The
assert
statement from Python will be used to write assertions. Thepytest
package includes some enhancements to theassert
statement to provide more verbose output when there is a failure. - The
pytest
test runner will be used to run the tests, as it is required to use the enhancedassert
. This test runner has full support for theTestCase
class from theunittest
package.
Don’t worry if some of these things don’t make much sense yet. The examples that are coming will make it more clear.
Testing a Fizz Buzz Application
The “Fizz Buzz” game consists in counting from 1 to 100, but replacing the numbers that are divisible by 3 with the word “Fizz”, the ones that are divisible by 5 with “Buzz”, and the ones that are divisible by both with “FizzBuzz”. This game is intended to help kids learn division, but has been made into a very popular coding interview question.
I googled for implementations of the “Fizz Buzz” problem in Python and this one came up first:
1 | for i in range(1, 101): |
After you’ve seen the forty_two()
unit test example above, testing this code seems awfully difficult, right? For starters there is no function to call from a unit test. And nothing is returned, the program just prints results to the screen, so how can you verify what is printed to the terminal?
To test this code in this original form you would need to write a functional test that runs it, captures the output, and then ensures it is correct. Instead of doing that, however, it is possible to refactor the application to make it more unit testing friendly. This is an important point that you should remember: if a piece of code proves difficult to test in an automated way, you should consider refactoring it so that testing becomes easier.
Here is a new version of the “Fizz Buzz” program above that is functionally equivalent but has a more robust structure that will lend better to writing tests for it:
1 | def fizzbuzz(i): |
What I did here is to encapsulate the main logic of the application in the fizzbuzz()
function. This function takes a number as input argument and returns what needs to be printed for that number, which can be Fizz
, Buzz
, FizzBuzz
or the number.
What’s left after that is the loop that iterates over the numbers. Instead of leaving that in the global scope I moved it into a main()
function, and then I added a standard top-level script check so that this function is automatically executed when the script is run directly, but not when it is imported by another script. This is necessary because the unit test will need to import this code.
I hope you now see that there is some hope and that testing the refactored code might be possible, after all.
Writing a test case
Since this is going to be a hands-on exercise, copy the refactored code from the previous section and save it to a file named fizzbuzz.py in an empty directory in your computer. Open a terminal or command prompt window and enter this directory. Set up a new Python virtual environment using your favorite tool.
Since you will be using pytest
in a little bit, install it in your virtual environment:
1 | (venv) $ pip install pytest |
The fizzbuzz()
function can be tested by feeding a few different numbers and asserting that the correct response is given for each one. To keep things nicely organized, separate unit tests can be written to test for “Fizz”, “Buzz” and “FizzBuzz” numbers.
Here is a TestCase
class that includes a method to test for “Fizz”:
1 | import unittest |
This has some similarities with the forty_two()
unit test, but now the test is a method within a class, not a function as before. The unittest
framework’s TestCase
class is used as a base class to the TestFizzBuzz
class. Organizing tests as methods of a test case class is useful to keep several related tests together. The benefits are not going to be evident with the simple application that is the testing subject in this article, so for now you’ll have to bear with me and trust me in that this makes it easier to write more complex unit tests.
Since testing for “Fizz” numbers can be done really quickly, the implementation of this test runs a few numbers instead of just one, so a loop is used to go through a list of several “Fizz” numbers and asserting that all of them are reported as such.
Save this code in a file named test_fizzbuzz.py in the same directory as the main fizzbuzz.py file, and then type pytest
in your terminal:
1 | (venv) $ pytest |
The pytest
command is smart and automatically detects unit tests. In general it will assume that any Python files named with the test_[something].py or [something]_test.py patterns contain unit tests. It will also look for files with this naming patterns in subdirectories. A common way to keep unit tests nicely organized in a larger project is to put them in a tests package, separately from the application source code.
If you want to see how does a test failure looks like, edit the list of numbers used in this test to include 4 or some other number that is not divisible by 3. Then run pytest
again:
1 | (venv) $ pytest |
Note how the test stopped as soon as one of the numbers failed to test as a “Fizz” number. To help you in figuring out exactly what part of the test failed, pytest
shows you the source code lines around the failure and the expected and actual results for the failed assertion. It also captures any output that the test prints and includes it in the report. Above you can see that the test went through numbers 3 and 4 and that’s when the assertion for 4 failed, causing the test to end. After you experiment with test failures revert the test to its original passing condition.
Now that you’ve seen how “Fizz” numbers are tested, it is easy to add two more unit tests for “Buzz” and “FizzBuzz” numbers:
1 | import unittest |
Running pytest
once again now shows that there are three unit tests and that all are passing:
1 | (venv) $ pytest |
Test Coverage
Are the three tests above good enough? What do you think?
While you are going to have to use your own judgement to decide how much automated testing you need to have confidence that your tests give adequate protection against failures in the future, there is one tool called code coverage that can help you get a better picture.
Code coverage is a technique that consists in watching the code as it executes in the interpreter and keeping track of which lines run and which do not. When code coverage is combined with unit tests, it can be used to get a report of all the lines of code that your unit tests did not exercise.
There is a plugin for pytest
called pytest-cov that adds code coverage support to a test run. Let’s install it into the virtual environment:
1 | (venv) $ pip install pytest-cov |
The command pytest --cov=fizzbuzz
runs the unit tests with code coverage tracking enabled for the fizzbuzz
module:
1 | (venv) $ pytest --cov=fizzbuzz |
Note that when running tests with code coverage it is useful to always limit coverage to the application module or package, which is passed as an argument to the --cov
option as seen above. If the scope is not restricted, then code coverage will apply to the entire Python process, which will include functions from the Python standard library and third-party dependencies, resulting in a very noisy report at the end.
With this report you know that the three unit tests cover 69% of the fizzbuzz.py code. I’m sure you agree that it would be useful to know exactly what parts of the application make up that other 31% of the code that the tests are currently missing, right? This could help you determine what other tests need to be written.
The pytest-cov
plugin can generate the final report in several formats. The one you’ve seen above is the most basic one, called term
because it is printed to the terminal. A variant of this report is called term-missing
, which adds the lines of code that were not covered:
1 | (venv) $ pytest --cov=fizzbuzz --cov-report=term-missing |
The term-missing
report shows the list of line numbers that did not execute during the tests. Lines 13 and 14 are the body of the main()
function, which were intentionally left out of the tests. Recall that when I refactored the original application I decided to split the logic into the main()
and fizzbuzz()
functions with the intention to have the core logic in fizzbuzz()
to make it easy to test. There is nothing in the current tests that attempts to run the main()
function, so it is expected those lines will appear as missing in terms of test coverage.
Likewise, line 18 is the last line of the application, which only runs when the fizzbuzz.py file is invoked as the main script, so it is also expected this line will not run during the tests.
Line 9, however, is inside the fizzbuzz()
function. It looks like one aspect of the logic in this function is not currently being tested. Can you see what it is? Line 9 is the last line of the function, which returns the input number after it was determined that the number isn’t divisible by 3 or by 5. This is an important case in this application, so a unit test should be added to check for numbers that are not “Fizz”, “Buzz” or “FizzBuzz”.
One detail that this report isn’t still being accurate about are lines that have conditionals in them. When you have a line with an if
statement such as lines 2, 4, 6 and 17 in fizzbuzz.py, saying that the line is covered does not give you the full picture, because these lines can execute in two very distinct ways based on the condition evaluating to True
or False
. The code coverage analysis can also be configured to treat lines with conditionals as needing double coverage to account for the two possible outcomes. This is called branch coverage and is enabled with the --cov-branch
option:
1 | (venv) $ pytest --cov=fizzbuzz --cov-report=term-missing --cov-branch |
Adding branch coverage has lowered the covered percentage to 65%. And the “Missing” column not only shows lines 9, 13, 14 and 18, but also adds those lines with conditionals that have been covered only for one of the two possible outcomes. The if
statement in line 17, which was reported as fully covered before, now appears as not been covered for the True
case, which would move on to line 18. And the elif
in line 6 is not covered for a False
condition, where execution would jump to line 9.
As mentioned above, a test is missing to cover numbers that are not divisible by 3 or 5. This is evident not only because line 9 is reported as missing, but also because of the missing 6->9
conditional. Let’s add a fourth unit test:
1 | import unittest |
Let’s run pytest
one more time to see how this new test helped improve coverage:
1 | (venv) $ pytest --cov=fizzbuzz --cov-report=term-missing --cov-branch |
This is looking much better. Coverage is now at 74%, and in particular all the lines that belong to the fizzbuzz()
function, which are the core logic of the application, are covered.
Code Coverage Exceptions
The four unit tests now do a good job at keeping the main logic tested, but the coverage report still shows lines 13, 14 and 18 as not covered, plus the conditional on line 17 as partially covered.
I’m sure you will agree that lines 17 and 18 are pretty safe, so it is an annoyance to have to see them listed in every coverage report. For cases where you as a developer make a conscious decision that a piece of code does not need to be tested, it is possible to mark these lines as an exception, and with that they will be counted as covered and will not appear in coverage reports as missing. This is done by adding a comment with the text pragma: no cover
to the line or lines in question. Here is the updated fizzbuzz.py with an exception made for lines 17 and 18:
1 | def fizzbuzz(i): |
Note how the comment was only added in line 17. This is because when an exception is added in a line that begins a control structure, it is applied to the whole code block.
Let’s run the tests one more time:
1 | (venv) $ pytest --cov=fizzbuzz --cov-report=term-missing --cov-branch |
This final report looks much cleaner. Should lines 13 and 14 also be marked as exempt from coverage? That is really up to you to decide. I’m always willing to exclude lines that I’m 100% sure I’ll never need to test, but I’m not really sure the main()
function in lines 13 and 14 falls into that category.
Writing a unit test for this function is going to be tricky because of the print()
statements, and it is definitely out of scope for this introductory article. It is not impossible to do it, though. My preference is to leave those lines alone, as a reminder that at some point I could figure out a good testing strategy for them. The alternative point of view would be to say that this is a piece of code that is stable and unlikely to change, so the return of investment for writing unit tests for it is very low, and in that case it would also be okay to exempt it from code coverage. If you add an exception for lines 13 and 14 then the coverage report will show 100% code coverage.
Conclusion
I hope this was a good introduction to unit testing in Python. In the following articles in the series I’ll be looking at testing more complex code. My intention is to be very thorough and cover many different types of applications and testing techniques. If you have a particular problem related to unit testing feel free to mention it to me in the comments so that I keep it in mind for future articles!
参考文献
[1]. How to Write Unit Tests in Python, Part 1: Fizz Buzz
[2]. 维基百科-单元测试
[3]. 维基百科-集成测试
若你觉得我的文章对你有帮助,欢迎点击上方按钮对我打赏
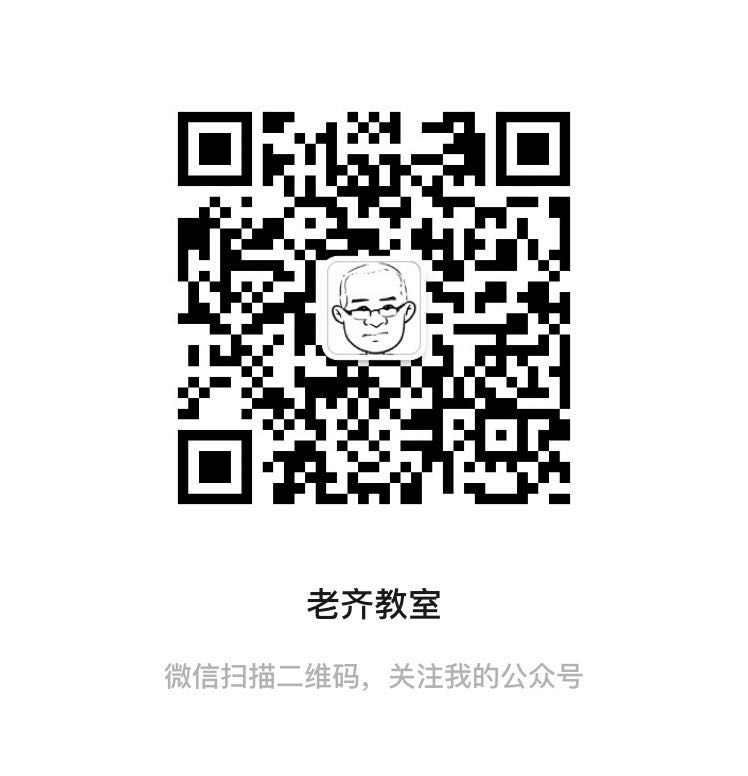
关注微信公众号,读文章、听课程,提升技能